Research: LKCMedicine scientist uncovers how the brain solves new problems
![]() | By Sarah Zulkifli, Science Writer, Communications and Outreach |
Neurons in the brain constantly transmit signals during problem-solving. While the functioning of neurons in solving familiar problems is well understood, less is known about the process when the brain encounters novel problems. One possible solution is to combine previously acquired knowledge and skills and apply them to new situations.
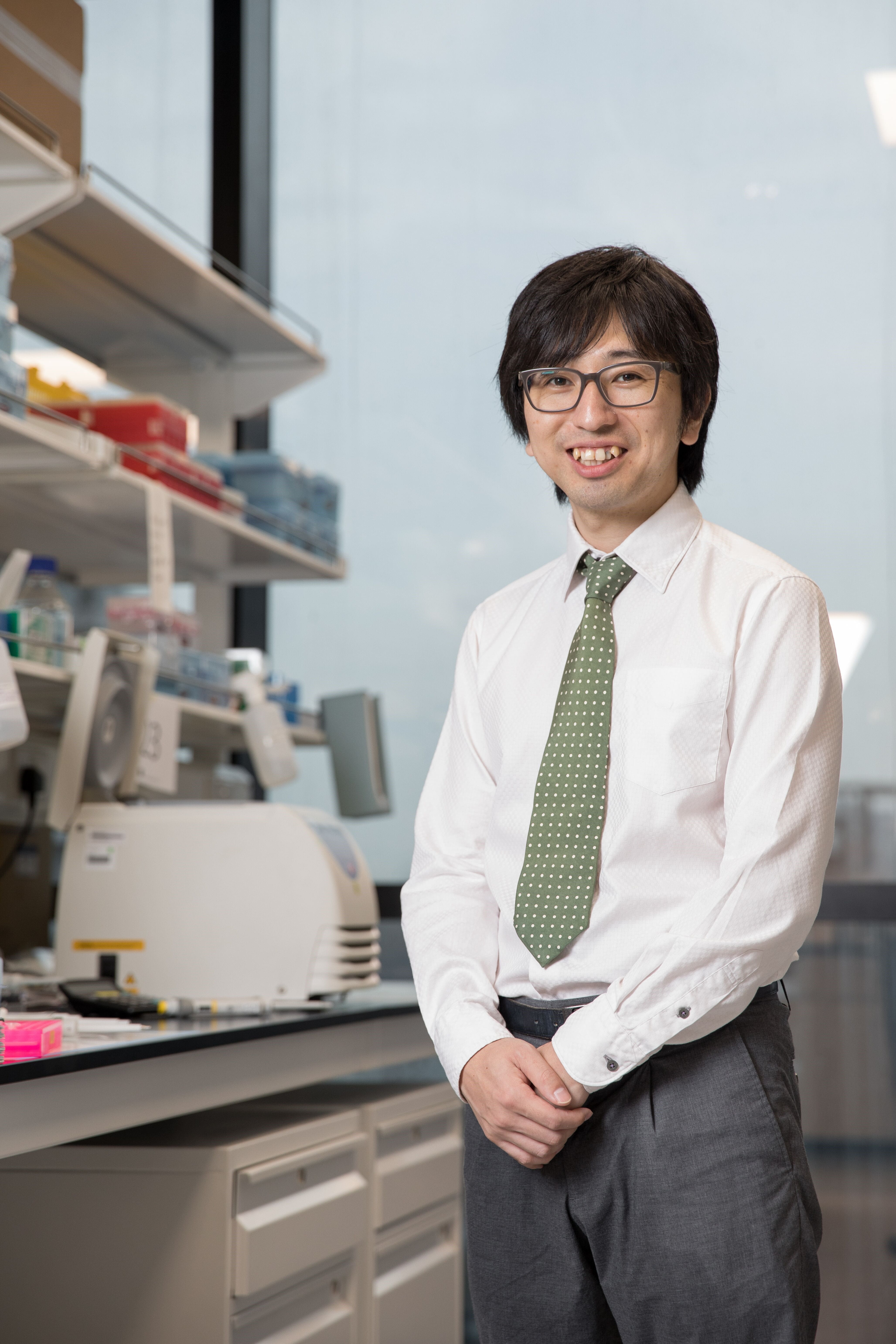
Nanyang Assistant Professor Hiroshi Makino
LKCMedicine Nanyang Assistant Professor Hiroshi Makino recently carried out a study exploring the possible similarities in how the brain and artificial agents learn to solve new tasks.
His findings, published in Nature Neuroscience last December, have interesting implications for both Artificial Intelligence (AI) and neuroscience research. He showed how neurons in the brain coordinate to compose new behavioural skills by utilising a previously acquired behaviour repertoire.
In his research, Asst Prof Makino first examined the neural activity patterns of an artificial agent trained to solve a novel problem using a deep reinforcement learning algorithm. He then compared them with those of a mouse brain solving the same problem.
Reinforcement learning is a sub-domain of machine learning. Its objective is to encourage an artificial agent to take a rewarding action and discouraging an undesired one. The artificial agent is able to perceive the environment and learn to form optimal decisions through trial and error[1].
In a previous interview, Asst Prof Makino said, "Existing studies in psychology showed that humans and non-human animals combine pre-learned skills to expand their behaviour repertoires. However, how the brain achieves this remains poorly understood. I was inspired by research in deep reinforcement learning – a subfield of AI research – studying the same problem, and empirically tested theoretical predictions derived from it by recording neural activity in the mouse brain."
Using two-photon calcium imaging, a technique that is used for imaging neural activity, he empirically tested theoretical predictions from deep reinforcement learning regarding how new behaviours are composed. The results showed that the brain employs similar mechanisms to those predicted by an AI algorithm, which involves combining value representations of previously acquired behavioural skills. The research also highlighted that the variability of skills to be combined was critical for successful behaviour composition.
Asst Prof Makino explained, "As the mice solved a new problem by combining pre-acquired skills and knowledge, activity was recorded from single neurons in their brains. The resulting neural activity was compared with theoretical models derived from deep reinforcement learning where a simple arithmetic operation was used to combine values of pre-learned behaviours.
“I found similar activity patterns between the artificial agent trained with a deep reinforcement learning algorithm and the brain. One of the major contributions of the study is the integration of neuroscience and deep reinforcement learning to identify a potential mechanism for how the brain composes a new behaviour.”
The findings are significant and suggest that when tackling new tasks or solving new problems, the mammalian brain composes a new behaviour through a simple combination of previously acquired action-value representations. Moreover, when behaviour variability was increased in the tasks to be combined, the study noted improved successful behaviour composition.
Commenting on the significance of the study, Professor George Augustine, Director of LKCMedicine’s Neuroscience & Mental Health Programme, said, “This is a landmark study that continues Hiroshi’s comparison of learning in machines and living brains. Here, he shows that learning in both systems can be achieved by combining the results of previously learned subtasks. Remarkably, neurons in the living nervous system encode the same learning-related information as in the artificial system; this is a major development for the fields of both neuroscience and artificial intelligence.
“Additionally, his results show that natural variability in behavioural responses enhances the ability of both mice and machines to learn, indicating the value of not always doing things exactly the same way every time.”
The impact of this study could pave the way for new studies linking neuroscientific observations to deep reinforcement learning research. It provides a new framework to study how various domains of biological intelligence are implemented in the brain. By learning how the brain works for problem-solving, AI researchers could come up with better algorithms and neural network architectures to improve machine intelligence.
Asst Prof Makino said, “A mechanistic understanding of how the brain combines pre-learned behaviour repertoires is important, because the concept can be extended to other disciplines of science, including combinatorial evolution of technology. Furthermore, addressing this question serves as a precedent of how to study neural mechanisms underlying other domains of biological intelligence.”
He added, “I now plan to study neural mechanisms of various domains of natural intelligence by applying theoretical concepts and tools of AI to neuroscience.”
Prof Augustine weighed in, “Hiroshi’s work is a huge contribution because it teaches us more about how we learn and which parts of the brain are involved in learning. This will have many downstream benefits for clinicians, such as neurologists and psychiatrists. It also shows us how similar our brains are to AI-based artificial systems. Finally, it also is helping to carve out a powerful new field – nestled between the AI and Neuroscience fields – that promises to deliver even more insights into the workings of our brain.
“This is one of the most significant scientific breakthroughs yet from our Neuroscience & Mental Health Programme. It is even more noteworthy because it is a rare, single-authored paper coming from one of our junior faculty members.”
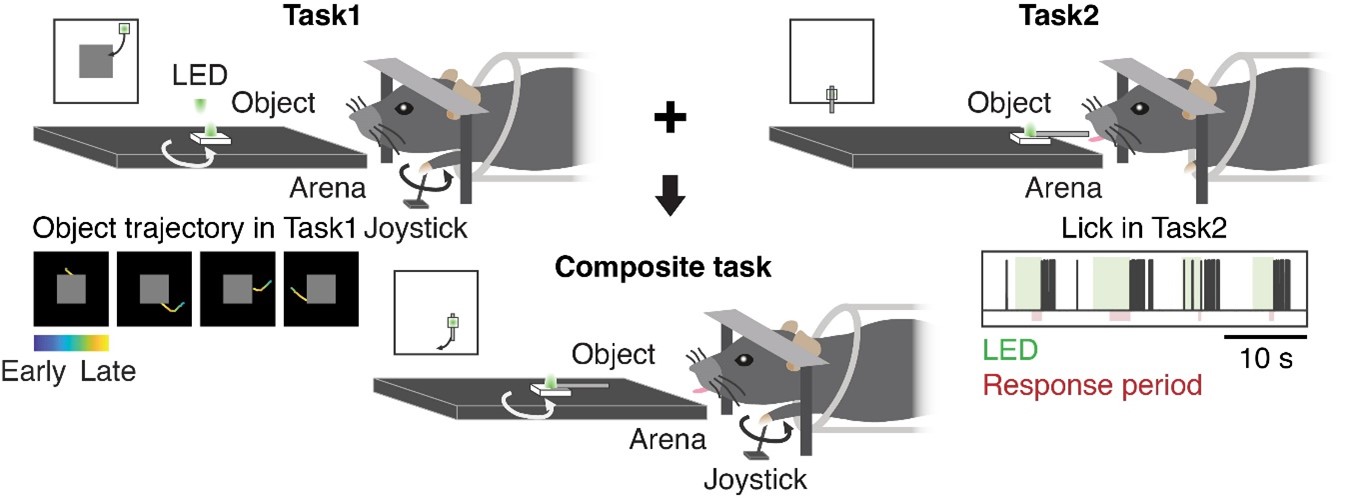
Hierarchical behaviour composition in mice
-