Low Resolution Information Also Matters: Learning Multi-Resolution Representations for Person Re-Identification
- New network design for multi-resolution person images and multi-resolution feature representation learning
Person re-identification (re-ID) is a retrieval task of recognizing the same person across images from non-overlapped cameras, which has attracted increasing attention in computer vision community due to its wide application prospects in video surveillance and forensics field. Nevertheless, person re-ID remains a challenge due to complicated visual variations in real scenarios such as viewpoint, illumination, person pose and background clutter. Captured images have variable resolutions affected by factors such as shooting distance and camera types, especially in real and unconstrained scenarios. The problem of matching person images with variable resolutions is defined as Cross-Resolution Person Re-ID. It is a key technique for intelligent monitoring systems practical for public security.
It is among the first works to explore the influence of resolution on feature extraction in person re- ID. To be specific, we have proposed a novel method named Multi-Resolution Representations Joint Learning (MRJL) for cross-resolution person re-ID, which fully utilizes features contained in different resolutions, and boosts the performance of cross-resolution person re-ID from two aspects:
- Accuracy (surpassing the state-of-the-art methods by a large margin)
- Robustness (performing robustly under the low-resolution condition)
The recent proposed methods for cross-resolution person re-ID mainly applied super-resolution (SR) technology to restore low-resolution (LR) images to high-resolution (HR) images. Although the complementary details generated by SR give person images better visual quality, these details may not be always reliable in person appearances. Therefore, in some cases, features extracted from these generated HR images are not discriminative enough to match correct persons. Although local details are lost in LR images, LR images still can provide reliable global information. These LR features can complement HR features which may have false details, but all existing methods neglect this useful information.
Our MRJL framework includes two parts:
- Resolution Reconstruction Network for generating multi-resolution person images
- Dual Feature Fusion Network for learning multi-resolution feature representations
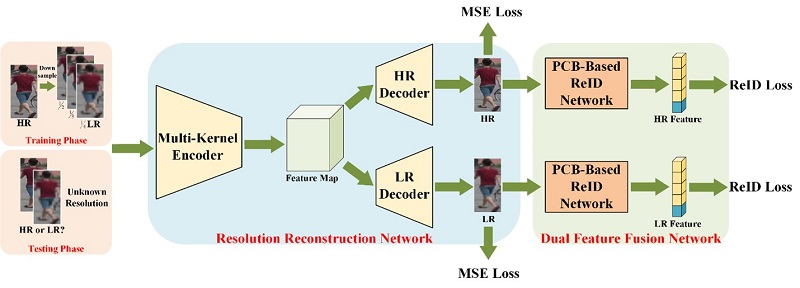
This method can be applied to person tracking in public monitoring systems, album management on intelligent mobile phones, and customer identification in unmanned supermarkets etc.
Guoqing Zhang, Yuhao Chen, Weisi Lin*, Arun Kumar Chandran, Jing Xuan, “Low Resolution Information Also Matters: Learning Multi-Resolution Representation for Person Re-identification”, Proceedings of the International Joint Conference on Artificial Intelligence (IJCAI), 2021, pp. 1295-1301. ( Link)