Introduction
The Anomaly Detection project is trying to tackle the problem of detecting anomalous events from surveillance video using non-rule-based approaches. It is an important and challenging problem with many surveillance applications, e.g., the detection of non-payment in subways. The researchers have proposed to describe both motion and appearance information with a new region based descriptor called “Motion Context” and formulate the abnormal event detection as a matching problem. The advantage of this scheme is that it is more robust than statistic model based methods, especially when the training dataset is of limited size.
Abnormal event detection systems are designed to detect abnormal human behaviors or events, based on non-rule based approaches, at public places from videos captured by surveillance cameras.The main purpose of such systems is to provide timely detection of potentially hazardous behaviors thus allowing early actions to be taken to prevent or respond to events such as accidents or wrong-doing. For example, the system should know to raise alerts when (i) a cyclist, a truck, or a skater runs into the pedestrian walkway, (ii) when a person passes the subway entrance without making a payment, or (iii) when a walking crowd suddenly runs away into different directions, which may indicate an explosion or a fire. The research team has developed advanced methods for detecting abnormal video events, which is general enough to be applied to both local and global events. They have also developed a highly accurate method for localizing abnormal events in videos with significant improvements over state-of-the-art methods.
Here is Abnormal behavior detection in crowded scenes, first two picture show detecting violations in the subway:

Then is detecting and localizing abnormal events on the pedestrian walkway:

Human activity detection is also of great interests for video surveillance systems. Current activity detection methods are not efficient enough to be applicable to large scale video databases. Therefore, to make such systems practical, the research team had developed novel methods for fast indexing and searching human activities in large-scale video databases. Their methods have achieved comparable accuracy to the state-of-the-art action detection and search methods but are significantly faster.
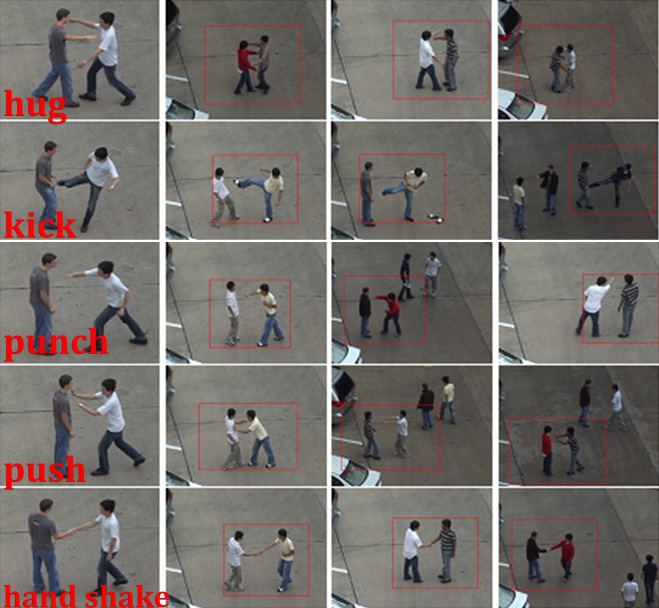
The Future
The Anomaly Detection project is trying to tackle the problem of detecting anomalous events from surveillance video using non-rule-based approaches. It is an important and challenging problem with many surveillance applications, e.g., the detection of non-payment in subways. The researchers have proposed to describe both motion and appearance information with a new region based descriptor called “Motion Context” and formulate the abnormal event detection as a matching problem. The advantage of this scheme is that it is more robust than statistic model based methods, especially when the training dataset is of limited size.
People Involved