Bio-Data Science and Education Group
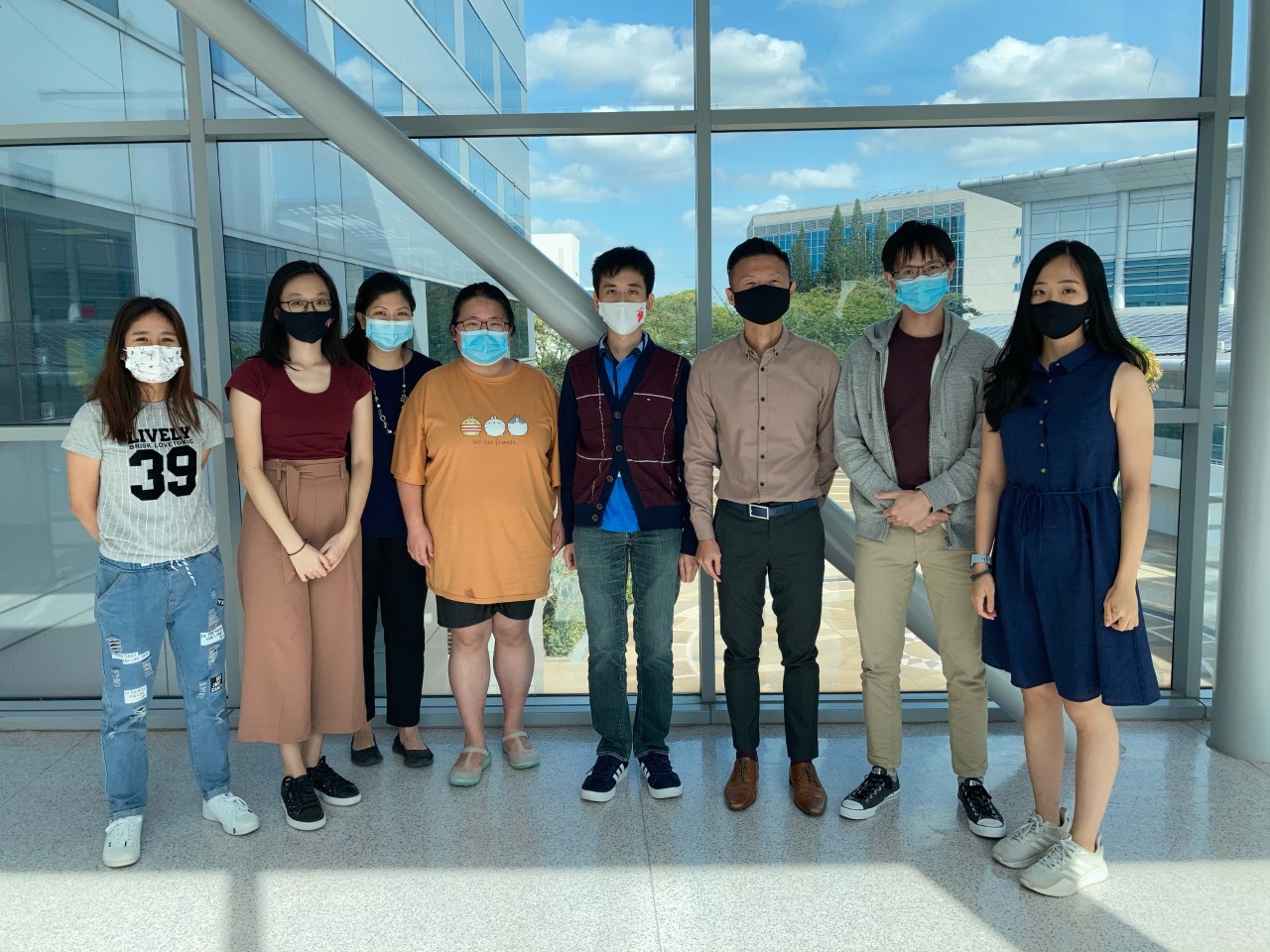
The Bio-Data Science laboratory is focused on the development of
statistical approaches for analysing and resolving platform-specific
idiosyncrasies in multi-omics data; identifying and resolving
confounding issues such as batch effects, technical bias and missing
values in high-dimensional data; and developing robust biomarker and
drug target prediction techniques using a combination of machine
learning and enhanced in silico validation techniques. This lab is also
interested in Bio-education, with an emphasis on the use of new AI-based
technologies, text-mining and high-impact pedagogical practices
(experiential learning), to enhance the quality of biological and
biotechnological education.
![]() | Lead PI
Wilson Goh Assistant Professor Email: [email protected] Phone: (65) 6904 7149 Office: Experimental Medicine Building (EMB) |
![]() | Allen Chong Senior Research Fellow Email: [email protected] |
![]() | Chan Wei Xin Research Fellow Email: [email protected] |
![]() | Peng Hui Research Fellow Email: [email protected] |
![]() | Stefano Perna Research Fellow Email: [email protected] |
![]() | Phua Ser Xian Research Assistant Email: [email protected] |
- Design and development of digital coaching environment for Deeper Experiential Engagement Projects: coevolution of education with Artificial Intelligence
- Engaging students as partners in the context of a scientific-thinking course with OBTL elements
- Identify Essential Genes to Construct A Functional Unfolded Protein Response Programme Using Synthetic Biology
- Evaluating the Clinical Utility of Immune Phenotypes in SchizophreniatIdes
- Goh WWB*, Wong LS. Turning straw into gold: how to build robustness into gene signature inference. Drug Discovery Today, 24(1):31-36, Jan 2019
- Goh WWB*, Sze CC. AI paradigms for teaching biotechnology. Trends in Biotechnology, 37(1): 1-5, Jan 2019
- Goh WWB*, Wong LS. Advanced bioinformatics methods for practical applications of proteomics. Briefings in Bioinformatics, 20(1):347-355, Jan 2019
- Goh WWB*, Wong LS. Breast Cancer Signatures Are No Better Than Random Signatures Explained. Drug Discovery Today, 23(11):1818-1823, Nov 2018
- Goh WWB*, Wong LS. Dealing with confounders in -omics analysis. Trends in Biotechnology, 36(5):488-498, May 2018
- Goh WWB*, Wong LS. Integrating networks and proteomics: moving forward. Trends in Biotechnology, 34(12):951-959, Dec 2017
- Goh WWB*, Sng J, Yee JY, See YM, Lee TS, Wong LS, Lee J. Can peripheral blood-derived gene expressions genetically characterize high risk subjects for psychosis? Computational Psychiatry, 0(1):1-16, Oct 2017
- Goh WWB*, Wong LS. NetProt: Complex-based feature selection. Journal of Proteome Research, 16(8):3102–3112, June 2017
- Goh WWB*, Wang W, Wong LS. Why batch effects matter in omics data, and how to avoid them. Trends in Biotechnology, S0167-7799(17)30036-7, Mar 2017
- Goh WWB*, Wong LS. Advancing clinical proteomics via networks: A tale of five paradigms. Journal of Proteome Research, 15(9):3167-3179, Jul 2016