Seminar on Data assimilation in flow and noise prediction applicable to green aerial transport
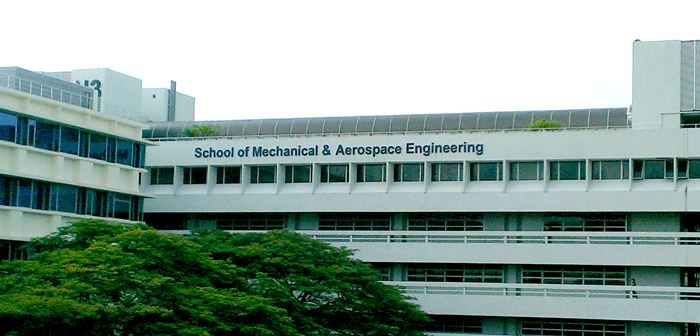
Dr Nick Zang Senior Lecturer in Aerospace Engineering Queen Mary University of London This seminar will be chaired by A/P Daniel New. |
Seminar Abstract |
Developing sustainable transport system is considered both a necessity and a significant drive for engineering innovations nowadays. As the research problems become increasingly complex – for instance, the next-generation urban air mobility vehicles often comprise multi-rotor configurations interacting with its operation environment – researchers are constantly looking for new toolsets to help better explore the design space and improve efficiency of these vehicles. Among them, machine learning is a promising enabler to such objectives. In this seminar, I would like to share our work on understanding the flow and aerodynamic noise of an electric propulsor interacting with turbulence, as the baseline configuration for green aerial vehicles. Subsequently, some preliminary developments and insights on how we could potentially assimilate data from different sources (e.g., experimental measurements, analytical formulae and numerical simulations) using a transfer/active learning framework to more accurately predict flow and noise of the different propulsor designs will be highlighted and discussed. |
Speaker's Biography |
Nick Zang is currently a Senior Lecturer in Aerospace Engineering at Queen Mary University of London (QMUL). Graduated from NTU, he mainly works in the intersection between fluid flows and aeroacoustics, that is to understand, predict and control aerodynamic noise based on insights from the physical flow mechanisms. Prior to joining QMUL, he has worked as a Lecturer at University of Bristol, where he has been pivotal to securing national funding for a new testbed for electric propulsion systems. He is also actively involved in the research and development of next-generation electric aircrafts as well as the applicable machine learning techniques, funded by InnovateUK, EPSRC and Horizon Europe. |