Seminar on Machine Learning and AI driven Process-Structure-Property Analytics and Decision-support for Additive Manufacturing
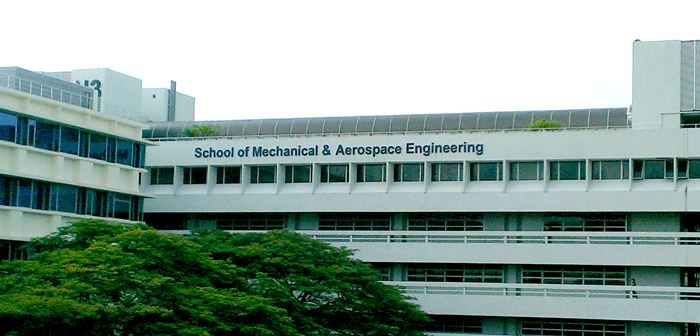
01 Apr 2021
10.30 AM - 11.30 AM
Current Students, Public
Dr. Hyunwoong KO National Institute of Standards and Technology (NIST), USA This seminar will be hosted by A/P Moon Seung Ki | |
Seminar Abstract | |
Additive manufacturing (AM) provides significantly improved manufacturing capabilities in terms of shape, material, hierarchical, and functional complexities. As a result, AM has the potential to revolutionize almost every industrial sector. However, despite this potential, there still is a lack of knowledge about AM’s dynamic and complex processes. To this end, my research has proposed a novel, Machine Learning (ML)-driven framework for learning Process-Structure-Property (PSP) knowledge and using the knowledge to support control-decisions for AM. The proposed framework consists of three tiers: knowledge of predictive models and physics, features of interest, and raw data. The framework defines a PSP-learning process with two sub-processes. The first uses a top-down, knowledge-graph-guided approach to generate the requirements for predictive analytics and data acquisition. The second uses a bottom-up, data-driven approach to construct new, PSP models. Together, these processes connect the framework to control-decisions and physical/virtual AM systems, respectively. In this seminar, I will introduce the proposed framework with a case study based on Laser Powder Bed Fusion processes including AM Metrology Testbed at National Institute of Standards and Technology (NIST). This study demonstrates the framework using NIST’s collaborative AM Material Database. The framework newly enables a systematic, hybrid, PSP-modeling approach for AM that can couple physics knowledge with the versatility of data-driven, ML models. Using the approach, the framework continuously updates the models (1) to improve understanding of dynamically generated AM data and (2) to link sub-models into coupled, PSP models. Based on the improved understanding, the framework facilitates control-decisions for AM at multiple scales. I will also discuss future research, which I have designed to further develop advanced manufacturing technologies. | |
Speaker’s Biography | |
|