Three Innovative Application of AI Awards from AAAI 2024
1. NTU + Shopee: Transformer-empowered Multi-modal Item Embedding for Enhanced Image Search in E-Commerce
Over the past decade, significant advances have been made in the field of image search for e-commerce applications. Traditional image-to-image retrieval models, which focus solely on image details such as texture, tend to overlook useful semantic information contained within the images. As a result, the retrieved products might possess similar image details, but fail to fulfil the user's search goals. Moreover, the use of image-to-image retrieval models for products containing multiple images results in significant online product feature storage overhead and complex mapping implementations. In this project, we design the Multi-modal Item EmbeddingModel (MIEM) to address these limitations. It is capable of utilizing both textual information and multiple images about a product to construct meaningful product features. By leveraging semantic information from images, MIEM effectively supplements the image search process, improving the overall accuracy of retrieval results. MIEM has become an integral part of the Shopee image search platform, with its features covering over 400 million products. Since its deployment in March 2023, it has achieved a remarkable 9.90% increase in terms of clicks per user and a 4.23% boost in terms of orders per user for the image search feature on the Shopee e-commerce platform.
SCSE Staff/Students Involved: NAP Yu Han, Dr Liu Chang (PhD graduate), Dr Zeng Anxiang (PhD graduate)
2. NTU + ENN Group: HiFi-Gas: Hierarchical Federated Learning Incentive Mechanism Enhanced Gas Usage Estimation
Gas usage estimation plays a critical role in various aspects of the power generation and delivery business, including budgeting, resource planning, and environmental preservation. Federated Learning (FL) has demonstrated its potential in enhancing the accuracy and reliability of gas usage estimation by enabling distributedly owned data to be leveraged, while ensuring privacy and confidentiality. However, to effectively motivate stakeholders to contribute their high-quality local data and computational resources for this purpose, incentive mechanism design is key. In this project, we design the Hierarchical FL Incentive mechanism for the Gas usage estimation (HiFi-Gas) system. It is designed to cater to the unique structure of gas companies and their affiliated heating stations. HiFi-Gas provides effective incentivization in a hierarchical federated learning framework that consists of a horizontal federated learning (HFL) component for effective collaboration among gas companies and multiple vertical federated learning (VFL) components for the gas company and its affiliated heating stations. To motivate active participation and ensure fairness among gas companies and heating stations, we incorporate a multi-dimensional contribution-aware reward distribution function that considers both data quality and model contributions. Since its deployment in the ENN Group in December 2022, HiFi-Gas has successfully provided incentives for gas companies and heating stations to actively participate in FL training, resulting in more than 12% higher average gas usage estimation accuracy and substantial gas procurement cost savings. This implementation marks the first successful deployment of a hierarchical FL incentive approach in the energy industry.
SCSE Staff/Students Involved: NAP Yu Han, Tang Xiaoli (PhD candidate)
3. NTU + Dareway Software: IBCA: An Intelligent Platform for Social Insurance Benefit Qualification Status Assessment
Social insurance benefits qualification assessment is an important task to ensure that retirees enjoy their benefits according to the regulations. It also plays a key role in curbing social security frauds. In this project, we develop the Intelligent Benefit Certification and Analysis (IBCA) platform, an AI-empowered platform for verifying the status of retirees to ensure proper disbursement of funds in Shandong province, China. Based on an improved Gated Recurrent Unit (GRU) neural network, IBCA aggregates missing value interpolation, temporal information, and global and local feature extraction to perform accurate retiree survival rate prediction. Based on the predicted results, a reliability assessment mechanism based on Variational Auto-Encoder (VAE) and Monte-Carlo Dropout (MC Dropout) is executed to perform reliability assessment. Deployed since November 2019, the IBCA platform has been adopted by 12 cities across the Shandong province, handling over 50 terabytes of data. It has empowered human resources and social services, civil affairs, and health care institutions to collaboratively provide high-quality public services. Under the IBCA platform, the efficiency of resources utilization as well as the accuracy of benefit qualification assessment have been significantly improved.
SCSE Staff/Students Involved: NAP Yu Han, Dr Shen Zhiqi, Prof Cyril Leung, Tang Xiaoli (PhD candidate)
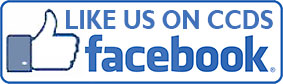
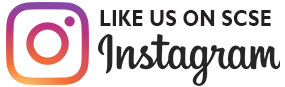
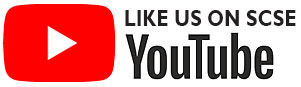